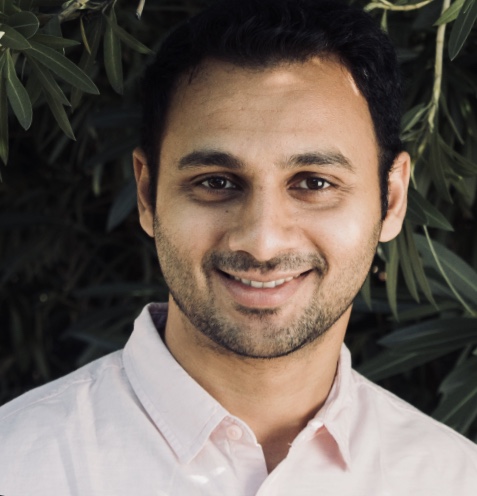
Hi! I am an applied scientist at Amazon AGI, working on customizing frontier models for downstream applications. Before this, I spent ~14 mo within the Ads / Sponsored Products org working on improving ad recommendations to drive higher shopper engagement and improve Amazon's retail business. Prior to Amazon, I spent 7.5 years at Lawrence Livermore National Laboratory as a Project Lead on foundational and applied ML problems.
Career Timeline
- Applied Science @ Amazon AGI (5/2025- present)
- Applied Science @ Amazon Ads (1/2024- 5/2025)
- Principal Scientist, Lawrence Livermore National Laboratory (10/2016-12/2023)
- Postdoc, IBM Research Almaden(4/2016-10/2016)
- PhD Student, Arizona State University (8/2010-3/2016)
(9/25/2024) Recent papers accepted for publication:
- [NeurIPS 2024] spotlight! On the Use of Anchoring for Training Vision Models (arXiv)
Explores short comings of anchoring for ViTs and fixes them to show significant improvements in ImageNet-1K OOD detection, calibration, generalization benchmarks. - [ICML 2024] PAGER: Accurate Failure Characterization in Deep Regression Models (openreview)
Failures in classifiers is clear (mis-classification rate) and well studied (OOD detection etc.), but very tricky in regression problems -- PAGER takes a step in formalizing failure analysis using a combination of epistemic and non-conformity scores - [ICLR 2024] Accurate and Scalable Estimation of Epistemic Uncertainty for Graph Neural Networks
(openreview)(webpage)
Introduces G-ΔUQ (pronounced "G-DUCK"), a novel training protocol for graph neural networks that supports reliable epistemic uncertainty estimates for graph classification and node classification tasks -- lot of SoTA results! - [IOP Machine Learning Sci & Tech] Transformer-Powered Surrogates Close the ICF Simulation-Experiment Gap with
Extremely Limited Data (article)
New training and model selection protocol for Transformer surrogates, which improve existing methods on few-shot adaption to real world ICF experiments by ~40% improvement in predictive performance.